
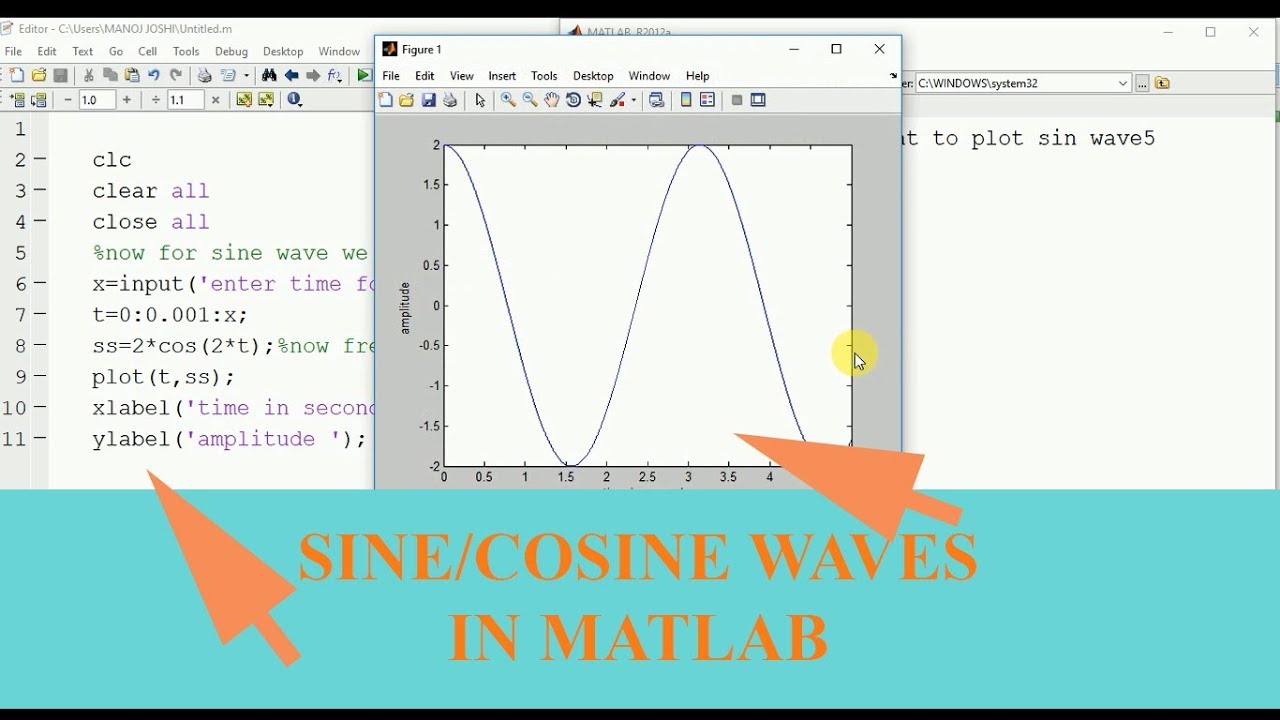
This algorithm has been concerned and studied by many scholars due to its simple implementation and less parameter setting, and its optimization search can be realized through simple variation of sine and cosine function values. Sine-cosine algorithm (SCA) is a new swarm intelligence optimization algorithm proposed by Mirjalili in 2016. At present, many swarm intelligence optimization algorithms are proposed like particle swarm optimization (PSO), differential evolution (DE), artificial bee colony algorithm (ABC), cuckoo search (CS), and flower pollination algorithm (FPA). So it is widely used in the engineering field of function optimization, combinatorial optimization, neural network training, and image processing. Compared with the traditional optimization methods, the swarm intelligence optimization algorithm is characterized by simple principle and fewer adjustment parameters, and the gradient information and strong global optimization algorithm of problems are not required. The swarm intelligence optimization algorithm is a kind of global optimization algorithm designed by simulating the mutual cooperation behavior mechanism of gregarious biology in nature. Accordingly, many scholars begin to explore new solution methods. These problems need to be continuous and derivable when the traditional methods are used for solving such practical engineering optimization problems, and these methods do not have the ability of global optimization for the multimodal, strong-nonlinearity, and dynamic change problems. The traditional methods which purely lie upon the exactly mathematical mode have unsatisfactory effect in solving such optimization problems. Many problems in the field of engineering practice and scientific research come down to the global optimization problems. The experimental results show that the proposed algorithm can effectively avoid falling into the local optimum, and it has faster convergence speed and higher optimization accuracy. Finally, the greedy Levy mutation strategy is used for the optimal individuals to enhance the local development ability of the algorithm. Secondly, it uses the random individuals near the optimal individuals to replace the optimal individuals in the primary algorithm, which allows the algorithm to easily jump out of the local optimum and increases the search range effectively. Firstly, the method of exponential decreasing conversion parameter and linear decreasing inertia weight is adopted to balance the global exploration and local development ability of the algorithm. The improvement involves three optimization strategies.
%2C+has+the+form%3A.jpg)
For the deficiency of the basic sine-cosine algorithm in dealing with global optimization problems such as the low solution precision and the slow convergence speed, a new improved sine-cosine algorithm is proposed in this paper.
